A new impetus for the use of PLS-SEM in business research
A Hungarian status report on the application of sem methodology, a glossary, and a breakdown of methodological barriers
DOI:
https://doi.org/10.14267/VEZTUD.2023.01.01Keywords:
research network, SEM, structural equation modellingAbstract
Structural equation modelling (SEM) is a popular multivariate analysis tool in marketing research that makes it possible to estimate both latent variables and their relationships. Variance-based (PLS) and covariance-based (CB) approaches are equal analysis methods based on recent methodological developments in PLS-SEM. The present study aims to eliminate the assumed methodological barriers of PLS-SEM and provide the domestic scientific community with a unified glossary and set of terms. The second part of the study examines SEM-related management articles published between 2016 and 2020. A distribution and thematic analysis revealed that the higher the prestige of the journal, the higher the SEM-publication ratio. Also, neither the chosen SEM methodology nor the analytics software were specified. Finally, a visualisation of research networks indicated that just a few scientific communities applied an SEM methodology in their research.
Downloads
References
Babin, B. J., Hair, J. F., & Boles, J. S. (2008). Publishing Research in Marketing Journals Using Structural Equation Modeling. Journal of Marketing Theory and Practice, 16(4), 279–286. https://doi.org/10.2753/MTP1069-6679160401
Benitez, J., Henseler, J., Castillo, A., & Schuberth, F. (2020). How to perform and report an impactful analysis using partial least squares: Guidelines for confirmatory and explanatory IS research. Information & Management, 57(2), 103168. https://doi.org/10.1016/j.im.2019.05.003
Beran, R., & Srivastava, M. (1985). Bootstrap tests and confidence regions for functions of a covariance matrix. The Annals of Statististics, 13(1), 95-115. https://doi.org/10.1214/aos/1176346579
Bollen, K. A. (1989). A New Incremental Fit Index for General Structural Equation Models. Sociological Methods & Research, 17(3), 303–316. https://doi.org/10.1177/0049124189017003004
Cole, D. A., Maxwell, S. E., Arvey, R., & Salas, E. (1993). Multivariate group comparisons of variable systems: MANOVA and structural equation modeling. Psychological Bulletin, 114(1), 174–184. https://doi.org/10.1037/0033-2909.114.1.174
Diamantopoulos, A., Riefler, P., & Roth, K. P. (2008). Advancing formative measurement models. Journal of Business Research, 61(12), 1203–1218. https://doi.org/10.1016/j.jbusres.2008.01.009
Dijkstra, T. K. (2017). A Perfect Match Between a Model and a Mode. In H. Latan & R. Noonan (Eds.), Partial Least Squares Path Modeling (pp. 55–80). Cham: Springer International Publishing. https://doi.org/10.1007/978-3-319-64069-3_4
Dijkstra, T. K., & Henseler, J. (2011). Linear indices in nonlinear structural equation models: Best fitting proper indices and other composites. Quality & Quantity, 45(6), 1505–1518. https://doi.org/10.1007/s11135-010-9359-z
Dijkstra, T. K., & Henseler, J. (2015a). Consistent and asymptotically normal PLS estimators for linear structural equations. Computational Statistics & Data Analysis, 81, 10–23. https://doi.org/10.1016/j.csda.2014.07.008
Dijkstra, T. K., & Henseler, J. (2015b). Consistent Partial Least Squares Path Modeling. MIS Quarterly, 39(2), 297–316. https://doi.org/10.25300/MISQ/2015/39.2.02
Fornell, C., & Larcker, D. F. (1981). Evaluating Structural Equation Models with Unobservable Variables and Measurement Error. Journal of Marketing Research, 18(1), 39-50. https://doi.org/10.2307/3151312
Franke, G., & Sarstedt, M. (2019). Heuristics versus statistics in discriminant validity testing: A comparison of four procedures. Internet Research, 29(3), 430–447. https://doi.org/10.1108/IntR-12-2017-0515
Füstös, L. (2009). A sokváltozós adatelemzés módszerei. Budapest: MTA Szociológiai Kutatóintézete Társadalomtudományi Elemzések Akadémiai Műhelye (TEAM). Guttman, L. (1945). A basis for analyzing test-retest reliability. Psychometrika, 10(4), 255–282. https://doi.org/10.1007/BF02288892
Hair, J. F., Hult, G. T. M., Ringle, C. M., Sarstedt, M., & Thiele, K. O. (2017). Mirror, mirror on the wall: A comparative evaluation of composite-based structural equation modeling methods. Journal of the Academy of Marketing Science, 45(5), 616–632. https://doi.org/10.1007/s11747-017-0517-x
Hair, J. F., Matthews, L. M., Matthews, R. L., & Sarstedt, M. (2017). PLS-SEM or CB-SEM: updated guidelines on which method to use. International Journal of Multivariate Data Analysis, 1(2), 107–134. https://doi.org/10.1504/IJMDA.2017.087624
Hair, J. F., Ringle, C. M., & Sarstedt, M. (2011). PLS-SEM: Indeed a Silver Bullet. Journal of Marketing Theory and Practice, 19(2), 139–152. https://doi.org/10.2753/MTP1069-6679190202
Hair, J. F., Sarstedt, M., Ringle, C. M., & Mena, J. A. (2012). An assessment of the use of partial least squares structural equation modeling in marketing research. Journal of the Academy of Marketing Science, 40(3), 414–433. https://doi.org/10.1007/s11747-011-0261-6
Hancock, G. R., Lawrence, F. R., & Nevitt, J. (2000). Type I Error and Power of Latent Mean Methods and MANOVA in Factorially Invariant and Noninvariant Latent Variable Systems. Structural Equation Modeling: A Multidisciplinary Journal, 7(4), 534–556. https://doi.org/10.1207/S15328007SEM0704_2
Henseler, J. (2012). Why generalized structured component analysis is not universally preferable to structural equation modeling. Journal of the Academy of Marketing Science, 40(3), 402–413. https://doi.org/10.1007/s11747-011-0298-6
Henseler, J. , Dijkstra, T. K., Sarstedt, M., Ringle, C. M., Diamantopoulos, A., Straub, D. W., Ketchen, D. J., Hair, J. F., Hult, G. T. M., & Calantone, R. J. (2014). Common Beliefs and Reality About PLS: Comments on Rönkkö and Evermann (2013). Organizational Research Methods, 17(2), 182–209. https://doi.org/10.1177/1094428114526928
Henseler, J. (2017). Bridging Design and Behavioral Research With Variance-Based Structural Equation Modeling. Journal of Advertising, 46(1), 178–192. https://doi.org/10.1080/00913367.2017.1281780
Henseler, J. (2018). Partial least squares path modeling: Quo vadis? Quality & Quantity, 52(1), 1–8. https://doi.org/10.1007/s11135-018-0689-6
Henseler, J. (2021). Composite-based structural equation modeling: Analyzing latent and emergent variables. London: The Guilford Press.
Henseler, J., Hubona, G., & Ray, P. A. (2016). Using PLS path modeling in new technology research: Updated guidelines. Industrial Management & Data Systems, 116(1), 2–20. https://doi.org/10.1108/IMDS-09-2015-0382
Henseler, J., Ringle, C. M., & Sarstedt, M. (2015). A new criterion for assessing discriminant validity in variance-based structural equation modeling. Journal of the Academy of Marketing Science, 43(1), 115–135. https://doi.org/10.1007/s11747-014-0403-8
Hwang, H., & Takane, Y. (2004). Generalized structured component analysis. Psychometrika, 69(1), 81–99. https://link.springer.com/content/pdf/10.1007/BF02295841.pdf
Iacobucci, D. (2009). Everything you always wanted to know about SEM (structural equations modeling) but were afraid to ask. Journal of Consumer Psychology, 19(4), 673–680. https://doi.org/10.1016/j.jcps.2009.09.002
Kazár K. (2014). A PLS-útelemzés és alkalmazása egy márkaközösség pszichológiai érzetének vizsgálatára. Statisztikai Szemle, 92(1), 35–52. https://www.ksh. hu/statszemle_archive/2014/2014_01/2014_01_033.pdf
Kemény I., Kulhavi N., & Kun Zs. (2022). A távorvoslás igénybevételét befolyásoló tényezők a COVID-19 járvány miatti félelem tükrében. Statisztikai Szemle, 100(1), 7-43. https://doi.org/10.20311/stat2022.1.hu0007
Nagy Á., Kemény I., Szűcs K., Simon J., & Kehl D. (2019). A véleményformáló magatartás mint másodrendű látens változó modellezése PLS-alapú strukturális egyenletek módszerével. Statisztikai Szemle, 97(9), 827–854. https://doi.org/10.20311/stat2019.9.hu0827
Reinartz, W., Haenlein, M., & Henseler, J. (2009). An empirical comparison of the efficacy of covariancebased and variance-based SEM. International Journal of Research in Marketing, 26(4), 332–344. https://doi.org/10.1016/j.ijresmar.2009.08.001
Rigdon, E. E. (2012). Rethinking Partial Least Squares Path Modeling: In Praise of Simple Methods. Long Range Planning, 45(5–6), 341–358. https://doi.org/10.1016/j.lrp.2012.09.010
Rigdon, E. E., Sarstedt, M., & Ringle, C. M. (2017). On Comparing Results from CB-SEM and PLS-SEM: Five Perspectives and Five Recommendations. Marketing ZFP, 39(3), 4–16. https://doi.org/10.15358/0344-1369-2017-3-4
Ringle, Sarstedt, & Straub. (2012). Editor’s Comments: A Critical Look at the Use of PLS-SEM in “MIS Quarterly.” MIS Quarterly, 36(1), iii-xiv. https://doi.org/10.2307/41410402
Sarstedt, M., Hair, J. F., Ringle, C. M., Thiele, K. O., & Gudergan, S. P. (2016). Estimation issues with PLS and CBSEM: Where the bias lies! Journal of Business Research, 69(10), 3998–4010. https://doi.org/10.1016/j.jbusres.2016.06.007
Sarstedt, M., Ringle, C. M., Henseler, J., & Hair, J. F. (2014). On the Emancipation of PLS-SEM: A Commentary on Rigdon (2012). Long Range Planning, 47(3), 154–160. https://doi.org/10.1016/j.lrp.2014.02.007
Schuberth, F., Henseler, J., & Dijkstra, T. K. (2018). Confirmatory Composite Analysis. Frontiers in Psychology, 9, 2541. https://doi.org/10.3389/fpsyg.2018.02541
Shmueli, G., Ray, S., Velasquez Estrada, J. M., & Chatla, S. B. (2016). The elephant in the room: Predictive performance of PLS models. Journal of Business Research, 69(10), 4552–4564. https://doi.org/10.1016/j.jbusres.2016.03.049
Sijtsma, K. (2009). On the Use, the Misuse, and the Very Limited Usefulness of Cronbach’s Alpha. Psychometrika, 74(1), 107–120. https://doi.org/10.1007/s11336-008-9101-0
Voorhees, C. M., Brady, M. K., Calantone, R., & Ramirez, E. (2016). Discriminant validity testing in marketing: An analysis, causes for concern, and proposed remedies. Journal of the Academy of Marketing Science, 44(1), 119–134. https://doi.org/10.1007/s11747-015-0455-4
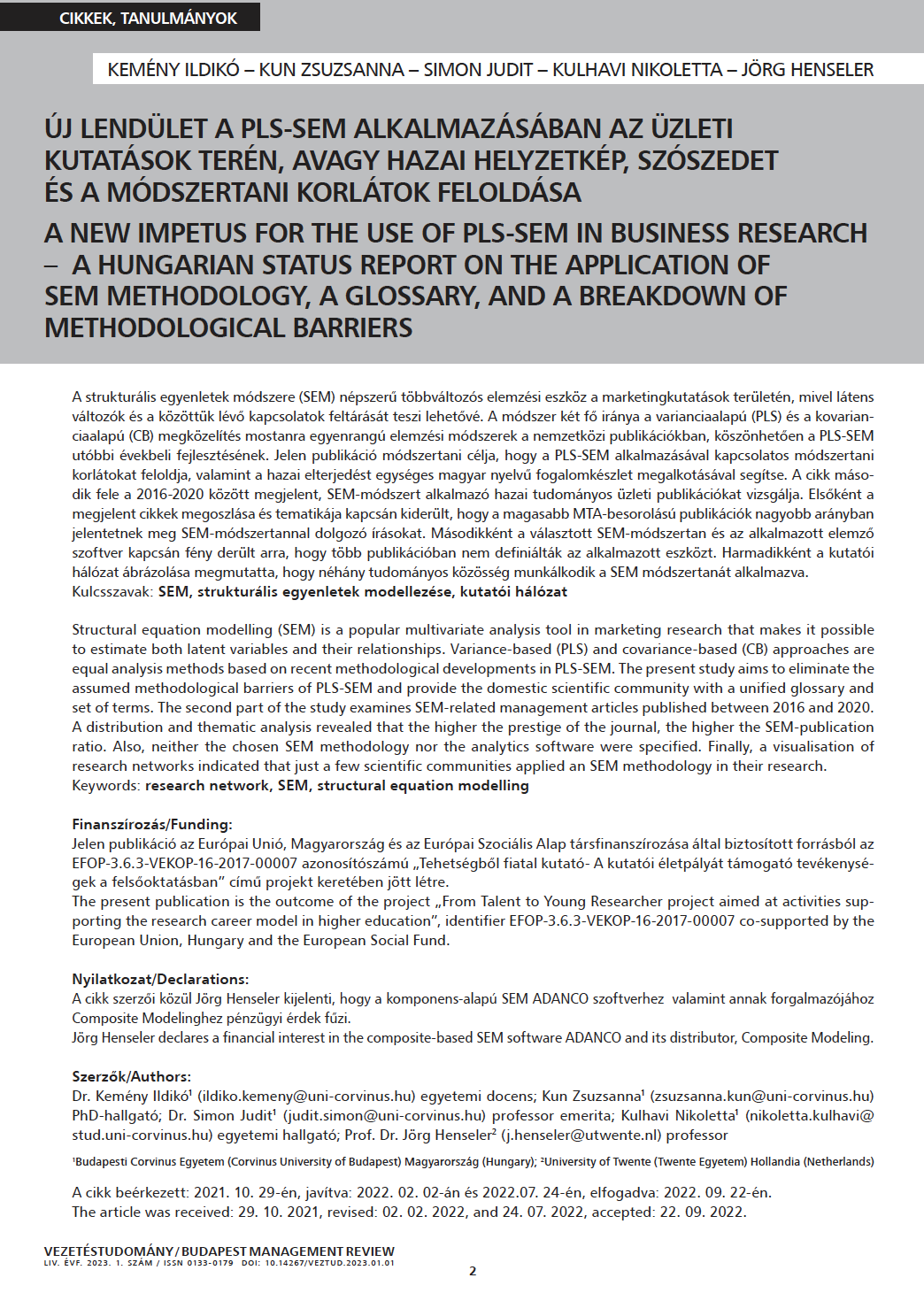
Downloads
Published
How to Cite
Issue
Section
License
Copyright (c) 2023 Vezetéstudomány / Budapest Management Review

This work is licensed under a Creative Commons Attribution 4.0 International License.
Authors assign copyright to Vezetéstudomány / Budapest Management Review. Authors are responsible for permission to reproduce copyright material from other sources.