ESG-szempontok a klímakockázat előrejelzésében
DOI:
https://doi.org/10.14267/VEZTUD.2021.09.02Kulcsszavak:
klímakockázat, neurális hálózat, fenntarthatóság, ESG-indikátorokAbsztrakt
Napjainkban a fenntarthatóság egyre nagyobb szerepet kap a pénzügyekben és ezzel együtt a klímakockázat mérése is előtérbe került. Kutatásuk során a szerzők az ESG-indikátorok (Environmental, Social and Governance) és a Global Climate Risk Index (CRI) kapcsolatát vizsgálták meg, fókuszálva a környezeti faktorok, valamint az országok jövedelmi kategóriájának szerepére. Elemzésükben a Világbank országszintű ESG-, valamint a Germanwatch klímakockázattal foglalkozó adatbázisait használták, amelyeken lineáris regresszió és neurális hálózat módszertant alkalmaztak a CRI előrejelzéséhez, amely az extrém időjárási események és a kapcsolódó társadalmi-gazdasági adatok következményeit számszerűsíti az emberáldozatok és a gazdasági veszteségek segítségével. A cikk fontos eredménye, hogy a klímakockázatot kevésbé jelzik előre a környezeti mutatók, inkább a társadalmi és kormányzati faktoroknak van jobb előrejelző képessége, illetve az országok jövedelmi szintje fordítottan arányos a klímaérzékenységgel. Eredményeik a nemzetközi és helyi politikai vezetésnek, valamint a befektetőknek lehetnek jelzésértékűek; minél alacsonyabb az ország jövedelmi helyzete, annál fokozottabb figyelmet kell fordítani az ESG-indikátorokra, ugyanis erősebben függnek össze a klímakockázattal.
Letöltések
Hivatkozások
Barr, R., Fankhauser, S., & Hamilton, K. (2010). Adaptation investments: A resource allocation framework. Mitigation & Adaptation Strategies for Global Change, 15(8), 843–858. https://doi.org/10.1007/s11027-010-9242-1
Berlinger, E., Keresztúri, J.,L., & Tamásné Vőneki, Zs. (2018). A cross-country analysis of operational risk: The effect of the freedom of press. In Dömötör Barbara (Ed.), PRMIA Hungary Chapter Éves Konferenciája, 2018: A Magyar kockázatkezelési kutatások legújabb eredményei (p. 8). Budapest: PRMIA Hungary.
Bertolotti, A. (2020). Effectively managing risks in an ESG portfolio. Journal of Risk Management in Financial Institutions, 13(3), 202–211.
Brooks, N., Neil Adger, W., & Mick Kelly, P. (2005). The determinants of vulnerability and adaptive capacity at the national level and the implications for adaptation. Global Environmental Change Part A: Human & Policy Dimensions, 15(2), 151–163. https://doi.org/10.1016/j.gloenvcha.2004.12.006
Busby, J. W., Smith, T. G., & Krishnan, N. (2014). Climate security vulnerability in Africa mapping 3.0. Political Geography, 43, 51–67. https://doi.org/10.1016/j.polgeo.2014.10.005
Choi, D., Gao, Z., & Jiang, W. (2020). Attention to global warming. The Review of Financial Studies, 33(3), 1112-1145. https://doi.org/10.2139/ssrn.3180045
Christiansen, N. H., Voie, P. E. T., Winther, O., & Høgsberg, J. (2014). Comparison of neural network error measures for simulation of slender marine structures. Journal of Applied Mathematics, 2014, Article ID 759834. https://doi.org/10.1155/2014/759834
Crabb, J. (2020). ESG: the financial costs of climate change. International Financial Law Review, 2020. https://www.iflr.com/article/b1lmx4n8t9626x/esg-the-financial-costs-of-climate-change
Eckstein, D., Künzel, V. & Schäfer L. (2017). Global Climate Risk Index 2018 [on-line]. Germanwatch. https://germanwatch.org/sites/germanwatch.org/files/publication/20432.pdf
Eckstein, D., Hutfils, M.-L, & Winges, M. (2018). Global Climate Risk Index 2019 [on-line]. Germanwatch. https://germanwatch.org/sites/germanwatch.org/files/Global%20Climate%20Risk%20Index%202019_2.pdf
Eckstein, D., Künzel, V., Schäfer L. & Winges, M. (2019). Global Climate Risk Index 2020 [on-line]. Germanwatch. https://www.germanwatch.org/sites/germanwatch.org/files/20-2-01e%20Global%20Climate%20Risk%20Index%202020_14.pdf
Eriksen, S., & Silva, J. A. (2009). The vulnerability context of a savanna area in Mozambique: Household drought coping strategies and responses to economic change. Environmental Science & Policy, 12(1), 33–52. https://doi.org/10.1016/j.envsci.2008.10.007
Friede, G., Busch, T., & Bassen, A. (2015). ESG and financial performance: Aggregated evidence from more than 2000 empirical studies. Journal of Sustainable Finance & Investment, 5(4), 210–233. https://doi.org/10.1080/20430795.2015.1118917
Glejser, H. (1969). A new test for heteroskedasticity. Journal of the American Statistical Association, 64(325), 316–323. https://doi.org/10.1080/01621459.1969.10500976
Griffin, P. A. (2020). Energy finance must account for extreme weather risk. Nature Energy, 5(2), 98–100. https://doi.org/10.1038/s41560-020-0548-2
Gu, S., Kelly, B., & Xiu, D. (2020). Empirical Asset Pricing via Machine Learning. Review of Financial Studies, 33(5), 2223–2273. https://doi.org/10.3386/w25398
Hale, J. (2020). Despite the Downturn, U.S. Sustainable Funds Notch a Record Quarter for Flows. https://www.morningstar.com/articles/977328/despite-the-downturn-us-sustainable-funds-notch-a-record-quarter-for-flows
Hong, H., Karolyi, G. A., & Scheinkman, J. A. (2020). Climate finance. The Review of Financial Studies, 33(3), 1011–1023. https://doi.org/10.2139/ssrn.3478737
IPCC. (2012). Managing the Risks of Extreme Events and Disasters to Advance Climate Change Adaptation [on-line]. New York: Cambridge University Press. https://www.ipcc.ch/site/assets/uploads/2018/03/SREX_Full_Report-1.pdf
IPCC (2014). Climate Change 2014 Impacts, Adaptation, and Vulnerability [on-line]. New York: Cambridge University Press. https://www.ipcc.ch/site/assets/uploads/2018/02/WGIIAR5-PartA_FINAL.pdf
Kahn, M. E. (2005). The Death Toll from Natural Disasters: The Role of Income, Geography, and Institutions. Review of Economics and Statistics, 87(2), 271–284. https://doi.org/10.2139/ssrn.391741
Kavzoglu, T., & Mather, P. M. (2003). The use of backpropagating artificial neural networks in land cover classification. International Journal of Remote Sensing, 24(23), 4907–4938. https://doi.org/10.1080/0143116031000114851
Kovács, E. (2014). Többváltozós adatelemzés. Budapest: Typotex.
Liu, C., Golding, D., & Gong, G. (2008). Farmers’ coping response to the low flows in the lower Yellow River: A case study of temporal dimensions of vulnerability. Global Environmental Change Part A: Human & Policy Dimensions, 18(4), 543–553. https://doi.org/10.1016/j.gloenvcha.2008.09.002
Matos, P. (2020). ESG and Responsible Institutional Investing Around the World: A Critical Review. SSRN Electronic Journal. https://doi.org/10.2139/ssrn.3668998
Monasterolo, I., Roventini, A., & Foxon, T. J. (2019). Uncertainty of climate policies and implications for economics and finance: An evolutionary economics approach. Ecological Economics, 163, 177–182. https://doi.org/10.1016/j.ecolecon.2019.05.012
Naffa, H., & Fain, M. (2020). Performance measurement of ESG-themed megatrend investments in global equity markets using pure factor portfolios methodology. PLoS ONE, 15(12), 1–34. https://doi.org/10.1371/journal.pone.0244225
Németh-Durkó, E. (2020). Környezet és pénzügyek: A pénzügyi fejlettség emissziót befolyásoló szerepe. Gazdaság és Pénzügy, 7(4), 434-449. https://doi.org/10.33926/gp.2020.4.4
Obersteiner, M., Azar, C., Kossmeier, S., Mechler, R., Moellersten, K., Nilsson, S., ... Yan, J. (2001). Managing climate risk. Science, 294(5545), 1282. https://doi.org/10.1126/science.294.5545.1282
Ostrom, E. (2009). A polycentric approach for coping with climate change. World Bank Policy Research Working Paper No. 5095. http://dx.doi.org/10.2139/ssrn.1934353
Prechelt, L. (1998). Automatic early stopping using cross validation: Quantifying the criteria. Neural Networks: The Official Journal of the International Neural Network Society, 11(4), 761–767. https://doi.org/10.1016/s0893-6080(98)00010-0
Primecz, H., Havran, D., & Lakatos, Z. (2019). How Does Female Presence on the Management and Supervisory Boards Impact the Performance in CEE? Academy of Management Annual Meeting Proceedings, 2019(1), 1–1. https://doi.org/10.5465/ambpp.2019.10602abstract
ROBECOSAM (2020). Country ESG Ranking Update – July 2020. Retrieved from https://www.robecosam.com/media/a/5/6/a565154e6efcd25e1197da40b69c1238_202008-robecosam-country-esg-ranking-robecosam_tcm1011-25282.pdf
Rubin, O., & Rossing, T. (2012). National and Local Vulnerability to Climate-Related Disasters in Latin America: The Role of Social Asset-Based Adaptation. Bulletin of Latin American Research, 31(1), 19–35. https://doi.org/10.1111/j.1470-9856.2011.00607.x
Sarkodie, S. A. (2018). The invisible hand and EKC hypothesis: What are the drivers of environmental degradation and pollution in Africa? Environmental Science and Pollution Research International, 25(22), 21993–22022. https://doi.org/10.1007/s11356-018-2347-x
Shive, S. A., & Forster, M. M. (2020). Corporate governance and pollution externalities of public and private firms. The Review of Financial Studies, 33(3), 1296-1330. https://doi.org/10.2139/ssrn.3339517
Tamásné Vőneki, Z., & Lamanda, G. (2020). Content analysis of bank disclosures related to ESG risks. Economy and Finance: English-Language Edition of Gazdaság és Pénzügy, 7(4), 412-424. https://doi.org/10.33908/EF.2020.4.3
Tato, A. & Nkambou R. (2018). Improving Adam Optimizer [online]. ICLR 2018 Workshop Submission. https://openreview.net/pdf?id=HJfpZq1DM
The World Bank (2019). Environment, Social and Governance Data [on-line]. New York: The Word Bank. https://datacatalog.worldbank.org/dataset/environment-social-and-governance-data
Thomas, V., Albert, J. R., & Perez, R. (2013). Climate-related disasters in Asia and the Pacific. Asian Development Bank Economics Working Paper Series, (358). Mandaluyong: Asian Development Bank. https://doi.org/10.2139/ssrn.2295783
Thomas, V. (2017). Climate change and natural disasters: transforming economies and policies for a sustainable future. New York: Routledge Taylor & Francis. https://doi.org/10.4324/9781315081045
Toya, H., & Skidmore, M. (2007). Economic Development and the Impacts of Natural Disasters. Economics Letters, 94(1), 20–25. https://doi.org/10.1016/j.econlet.2006.06.020
UNEP (2016). The Adaptation Finance Gap Report 2016 [online]. New York: United Nations Environment Programme (UNEP). https://climateanalytics.org/media/agr2016.pdf
van Dijk, M. A. (2020). Assessing climate risk for investment portfolios. Rotterdam: Rotterdam School of Management, Erasmus University. https://www.rsm.nl/fileadmin/Images_NEW/Erasmus_Platform_ for_Sustainable_Value_Creation/Assessing_climate_ risk_for_investment_portfolios__oct20.pdf
Volz, U., Beirne, J., Ambrosio Preudhomme, N., Fenton, A., Mazzacurati, E., Renzhi, N., & Stampe, J. (2020). Climate change and sovereign risk. London: SOAS Centre for Sustainable Finance, SOAS University of London. https://eprints.soas.ac.uk/33524/1/Climate%20Change%20and%20Sovereign%20Risk_final.pdf
Wang, Y., Li, Y., Song, Y., & Rong, X. (2020). The influence of the activation function in a convolution neural network model of facial expression recognition. Applied Sciences, 10(5), 1897. https://doi.org/10.3390/app10051897
Wheeler, D. (2011). Quantifying vulnerability to climate change: Implications for adaptation assistance. Washington, D.C.: Center for Global Development. https://doi.org/10.2139/ssrn.1824611
WHO (2011). The Social Dimensions of Climate Change [online]. World Health Organization (WHO). https://www.who.int/globalchange/mediacentre/events/2011/social-dimensions-of-climate-change.pdf
World Economic Forum (2020). The Global Risks Report 2020 [online]. http://www3.weforum.org/docs/WEF_Global_Risk_Report_2020.pdf
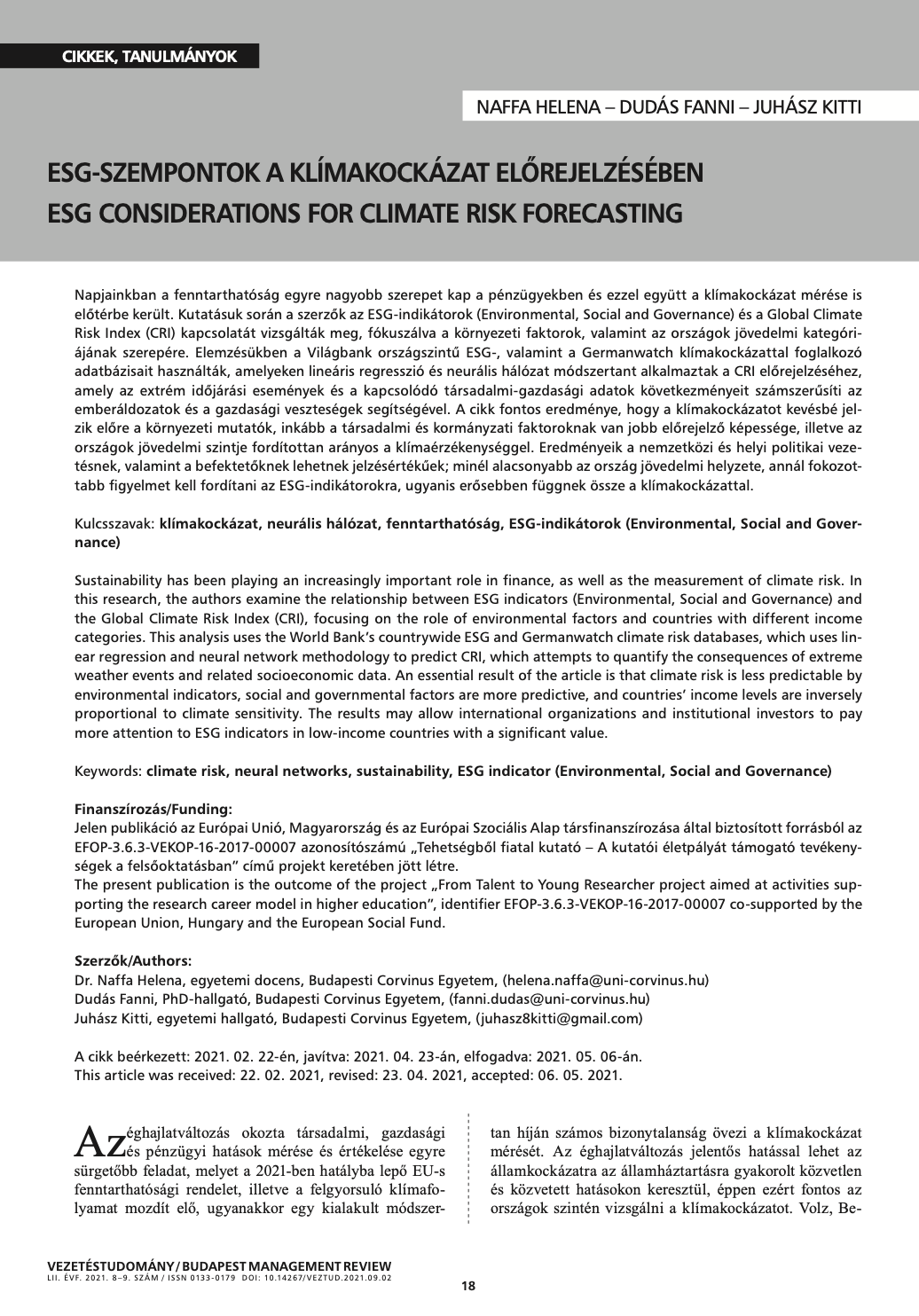
Downloads
Megjelent
Hogyan kell idézni
Folyóirat szám
Rovat
License
Copyright (c) 2021 Vezetéstudomány / Budapest Management Review

This work is licensed under a Creative Commons Attribution 4.0 International License.
Authors assign copyright to Vezetéstudomány / Budapest Management Review. Authors are responsible for permission to reproduce copyright material from other sources.